Protein pKa prediction
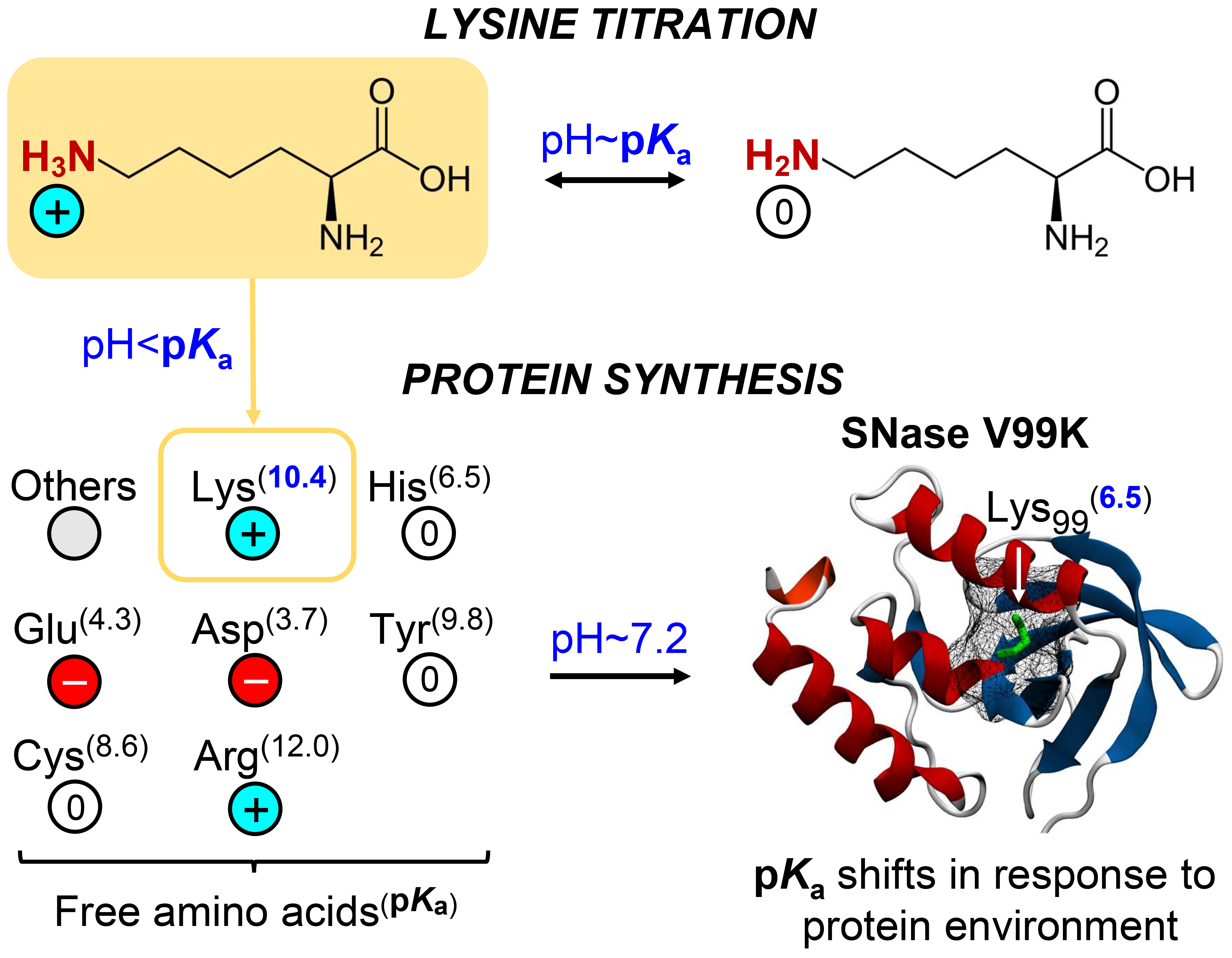
We developed, so far as we know, the first deep-learning based protein pKa predictor DeepKa, which is established by the data sets generated by continuous constant pH molecular dynamics simulations of 279 soluble proteins that include 12809 pKa values of four residue types, namely Asp, Glu, His and Lys. Notably, to avoid discontinuities at the boundary, grid charges are proposed to represent protein electrostatics. We show that the prediction accuracy by DeepKa is close to that by CpHMD benchmarking simulations, validating DeepKa as an efficient protein pKa predictor. In addition, the training and validation sets created in this study can be applied to the development of machine learning-based protein pKa predictors in the future. Finally, the grid charge representation is general and applicable to other topics, such as the protein-ligand binding affinity prediction. (Data and code can be downloaded for free from https://gitlab.com/yandonghuang/deepka.)